; Date: Sat May 12 2018
Tags: Node.js »»»» Asynchronous Programming
While async functions in JavaScript are a gift from heaven, application performance can suffer if they're used badly. The straightforward approach in using async functions in some cases makes performance go down the drain. Some smart recoding can correct the problem, unfortunately at the cost of code clarity.
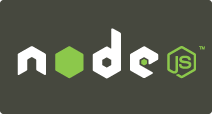
Consider the case of a sequence of async function invocations. You want to handle all invocations before proceeding to the next step. The question is the method with the cleanest code that also performs well.
Prototype problem & solution
A naive approach might do as so:
const fs = require('fs').promises;
const path = require('path');
const num2copy = process.env.PERF_NUM_COPY;
const copy2dir = process.env.PERF_DIRNM;
async function copyFile(src, dest) {
await fs.copyFile(src, dest);
}
(async () => {
let count = 0;
while (count < num2copy) {
let destfile = path.join(copy2dir, `datafile${count}.txt`);
console.log(`COPYING ${destfile}`);
await copyFile('srcfile.txt', destfile);
count++;
}
})();
We have an async function, copyFile
, standing in for any kind of asyncronous operation. The main action is the loop at the bottom, which copies the files one-by-one, waiting for each copy operation to finish before starting the next.
The code is extremely clean and easy to read and the programmers intention shines clearly. But, the files are copied .. One .. At .. A .. Time. That fact loses the opportunity to have interleaving code execution by running the operations simultaneously. There is no dependency between copyFile
invocations, and they would not interfer with each other.
If it's not clear, this example requires Node.js 10.1 because it uses the new fs.promises
API. If you want to run on a previous release, substitute the 3rd party fs-extra
module.
Consider a differrent implementation:
const parallelLimit = require('run-parallel-limit');
const fs = require('fs').promises;
const path = require('path');
const num2copy = process.env.PERF_NUM_COPY;
const copy2dir = process.env.PERF_DIRNM;
const numparallel = Math.floor(process.env.PERF_PARALLEL);
async function copyFile(src, dest) {
await fs.copyFile(src, dest);
}
(async () => {
const tasks = [];
let count = 0;
while (count < num2copy) {
let destfile = path.join(copy2dir, `datafile${count}.txt`);
tasks.push((cb) => {
console.log(`COPYING ${destfile}`);
copyFile('srcfile.txt', destfile)
.then(results => { cb(undefined, results) })
.catch(err => { cb(err); });
});
count++;
}
console.log(`num2copy ${num2copy} tasks ${tasks.length}`);
await new Promise((resolve, reject) => {
parallelLimit(tasks, numparallel, function(err, results) {
// gets here on final results
if (err) reject(err);
else resolve(results);
});
});
})();
This creates an array of functions that will call copyFile
- and it then uses parallelLimit
to run the tasks in parallel. By using this function we can specify the degree of concurrency.
It is possible to instead use this:
await Promise.all(tasks);
What would happen in this case is that all tasks
entries would start at the same time. What if your tasks array has thousands of entries? Would your application survive if all the thousands of tasks were to start at once? Using parallelLimit
keeps the simultaneity in check (I hope that's a word) so your application doesn't blow up.
Inspiration -- solving performance problems in AkashaCMS
Rendering techsparx.com
using
AkashaCMS was taking well over 1 hour 20 minutes. AkashaCMS is a static website generator system that I've developed over the last few years. This website has several hundred pages many of which include YouTube videos, and it seems that retrieving metadata from YouTube bogs down the rendering process.
My first stage of improvement was to buy a faster computer for rendering my websites and other tasks. I had an older Celeron-based Intel NUC with 8GB memory that was used to run Gogs (for Github-like service) and Jenkins (the continuous integration system). I push new website content to a repository on the NUC, and Jenkins is configured to automatically wake up and render the website. It was nice to just write content and have the system automatically take care of things. But as I added content, the time to render grew and grew.
The new NUC has Core i5 processor, 18GB memory, and is therefore a much faster computer. Rendering techsparx.com
dropped to 40-45 minutes, much better but still slow.
Then, I had an inspired thought ... which is explained in the previous section.
In AkashaCMS the processing is far more complex than that simple file copy. There are templates to render, custom tags to process, lots of YouTube URL's to retrieve metadata for, and so on.
But - as complex as the rendering process is, it fell to one function in render.js
to sequence the rendering. Turns out I'd written that function as a really nice and easy to read async loop, which processed the files one-at-a-time.
I thought, what if I were to rewrite that loop to render N files at a time? The result was a new rendering loop somewhat like the second example above. And, a massive performance gain.
Rendering techsparx.com
involves processing many hundreds of files and it didn't take much thought to realize the problem I named earlier. Rendering the entire site simultaneously using Promise.all
would have blown something up. Instead this required constrained concurrency.
For the code difference see:
github.com akashacms akasharender compare
Nice hand-waiving, lets see some numbers
It's one thing to wave your hands and make a claim. It's another thing to back it up with numbers. I have two sets of numbers to report, one using the above two applications, and one using AkashaCMS to render techsparx.com
.
First, we need an input file to copy. Read the code above and you'll see it makes N copies of an input file. It's an artificial benchmark, chosen to be somewhat similar to AkashaCMS's rendering loop.
Make a dummy file of 1GB size -- this was executed on a Linux box (my rendering NUC). For macOS the invocation is slightly different.
$ dd if=/dev/urandom of=srcfile.txt bs=64M count=16 iflag=fullblock
16+0 records in
16+0 records out
1073741824 bytes (1.1 GB, 1.0 GiB) copied, 28.7961 s, 37.3 MB/s
Then we run the first application to establish a baseline:
$ mkdir -p d
$ rm -rf d/* ; time PERF_DIRNM=`pwd`/d PERF_NUM_COPY=20 node perftest.js
COPYING /home/david/perftest/d/datafile0.txt
(node:20165) ExperimentalWarning: The fs.promises API is experimental
COPYING /home/david/perftest/d/datafile1.txt
COPYING /home/david/perftest/d/datafile2.txt
COPYING /home/david/perftest/d/datafile3.txt
COPYING /home/david/perftest/d/datafile4.txt
COPYING /home/david/perftest/d/datafile5.txt
COPYING /home/david/perftest/d/datafile6.txt
COPYING /home/david/perftest/d/datafile7.txt
COPYING /home/david/perftest/d/datafile8.txt
COPYING /home/david/perftest/d/datafile9.txt
COPYING /home/david/perftest/d/datafile10.txt
COPYING /home/david/perftest/d/datafile11.txt
COPYING /home/david/perftest/d/datafile12.txt
COPYING /home/david/perftest/d/datafile13.txt
COPYING /home/david/perftest/d/datafile14.txt
COPYING /home/david/perftest/d/datafile15.txt
COPYING /home/david/perftest/d/datafile16.txt
COPYING /home/david/perftest/d/datafile17.txt
COPYING /home/david/perftest/d/datafile18.txt
COPYING /home/david/perftest/d/datafile19.txt
real 9m2.562s
user 0m2.498s
sys 0m26.417s
We're making 20 copies of the file and it takes about 9 minutes to execute sequentially.
For the subsequent runs the command-line is:
$ rm -rf d/* ; time PERF_DIRNM=`pwd`/d PERF_NUM_COPY=20 PERF_PARALLEL=2 node perftest2.js
The table of results:
Concurrency | real | user | sys |
---|---|---|---|
2 | 5m52.841s | 0m1.004s | 0m28.086s |
3 | 6m21.449s | 0m1.052s | 0m27.989s |
4 | 8m9.749s | 0m0.878s | 0m30.790s |
5 | 7m50.680s | 0m0.776s | 0m29.847s |
6 | 5m35.472s | 0m0.661s | 0m27.840s |
7 | 5m54.364s | 0m0.714s | 0m28.648s |
8 | 5m38.746s | 0m0.720s | 0m29.185s |
9 | 5m51.299s | 0m0.689s | 0m28.813s |
10 | 7m19.212s | 0m1.252s | 0m29.907s |
11 | 9m3.156s | 0m0.618s | 0m32.067s |
11 | 8m1.227s | 0m1.043s | 0m30.161s |
11 | 5m34.230s | 0m1.059s | 0m28.115s |
11 | 5m40.371s | 0m0.766s | 0m29.142s |
I wouldn't take the specific numbers as gods given most accurate performance measurements. There's some variation if you run the same test multiple times. I ran with concurrency=11
four times to demonstrate that behavior.
However, the trend is that concurrency causes execution time to cut in half, approximately. In this case there isn't much difference between any level of concurrency.
The next example was to render techsparx.com
with different concurrency settings. You, the patient reader, will not be able to replicate this since you don't have the source code for techsparx.com
so you'll have to take my word for the following table.
Concurrency | real | user | sys |
---|---|---|---|
1 | 45m57.670s | 13m50.561s | 0m41.197s |
2 | 20m55.243s | 13m20.353s | 0m35.821s |
3 | 16m1.700s | 12m25.354s | 0m31.939s |
4 | 16m39.358s | 13m6.293s | 0m30.883s |
5 | 15m3.506s | 12m35.197s | 0m29.757s |
6 | 14m17.362s | 12m42.524s | 0m28.053s |
7 | 13m8.556s | 12m17.924s | 0m27.122s |
8 | 14m1.350s | 12m46.572s | 0m26.166s |
9 | 12m48.809s | 12m37.150s | 0m25.969s |
10 | 12m27.071s | 12m26.288s | 0m25.814s |
11 | 11m36.765s | 12m3.887s | 0m25.071s |
12 | 11m29.905s | 12m1.079s | 0m24.928s |
13 | 12m3.213s | 12m22.917s | 0m24.878s |
14 | 11m38.163s | 12m22.178s | 0m23.863s |
15 | 11m40.029s | 12m15.376s | 0m24.972s |
16 | 11m45.688s | 12m2.554s | 0m25.401s |
Clearly a huge improvement - from 45+ minutes down to about 12 minutes. I'll take that any day of the week.
Further gains to come
Another easy-to-imagine performance gain is to cache YouTube results in a local database rather than querying over and over for the same data. I'm sure YouTube's servers are getting tired of telling me about the same videos every few hours.